Could These Bones Be Amelia Earhart's? (Modeling Module)
Author: Marsha Davis
In this module, students create models for predicting human stature from bone lengths. The goal for the development of these models is to determine whether a set of bones found on Nikumaroro Island might be Amelia Earhart's. In Activity 1, students work with a model developed by Dr. Mildred Trotter for predicting height from tibia length. Since students will not be able to directly measure their own bone lengths, they collect height and forearm length measurements from the class. They compare heights of male students to female students. In Activity 2, students make a scatterplot of height versus forearm length from the class data. Based on their scatterplot, they discuss the direction, form, and strength of the relationship between height and forearm length. Then they create their first model by drawing a line (by hand) on their height-forearm scatterplot that they think summarizes the pattern in the data.
In Activity 3, students are given data from the Forensic Anthropology Data Bank at the University of Tennessee on height and bone lengths. In order to investigate the direction and form of the height-radius data, students use technology to make a scatterplot of height versus radius length. After sharing their Activity 2 models for predicting height from forearm length and discovering that different students created different models, students are introduced to the leastsquares criterion for picking the "best" model. Then they fit a leastsquares (regression) line to the data and use this model to predict the height of the person whose bones (including a radius) were found on Nikumaroro Island.
In Activity 4, students are introduced to the coefficient of determination, r2, a measure of the strength of a linear relationship. They determine leastsquares equations for the relationships between:
(1) height and radius,
(2) height and tibia, and
(3) height and arm (arm= radius + ulna)
Then they use r2 to select which of these relationships is the strongest. Using their chosen model, students use the bone lengths found on Nikumaroro Island to determine whether the bones might be Amelia Earhart's.
This module was adapted from Chapter 3 of Mathematics: Modeling Our World, Course 1, 2nd edition (2010), COMAP, Bedford MA.
Note: The information below was created with the assistance of AI.
Level of Mathematics
This module is applicable at a high school or early college level. It involves concepts such as linear regression, residual errors, sum of squared errors (SSE), and correlation coefficients, which are typically covered in advanced algebra or statistics courses.
Application Areas
1. Statistics: The content deals with statistical methods for data analysis.
2. Linear Regression: Students use linear models to predict heights based on bone lengths.
3. Data Analysis: Students collect and analyze data from various sources (e.g., internet, historical records).
Prerequisites
To understand the material in this module, students should have a solid foundation in:
1. Basic algebra: Understanding of equations, functions, and graphing.
2. Statistics: Familiarity with basic statistical concepts such as mean, median, mode, variance, and standard deviation.
3. Data handling: Ability to collect, organize, and interpret data.
Subject Matter
The subject matter primarily focuses on:
1. Linear Regression: Understanding how linear models can be used for prediction.
2. Residual Analysis: Calculating and interpreting residual errors in regression analysis.
3. Correlation Coefficient (r): Using the correlation coefficient to measure the strength of a linear relationship.
Correlation to Mathematics Standards
The content aligns with several mathematics standards, including:
1. CCSS.MATH.CONTENT.HS.S-IC.B.4: Use data inferences and predictions.
2. CCSS.MATH.CONTENT.HS.S-ID.C.7: Interpret the slope (rate of change) and intercept (constant term) of a linear model in terms of the context.
3. CCSS.MATH.CONTENT.HS.S-ID.C.8: Compute (using technology) and interpret the correlation coefficient of a linear fit.
4. CCSS.MATH.CONTENT.HS.S-IC.B.5: Recognize random variation from sample to sample, taking samples using different methods.
Summary
This module is designed for students at a high school or early college level, focusing on statistical analysis and linear regression. It requires a solid understanding of algebra and basic statistics. The content aligns with several mathematics standards related to data analysis and regression models.
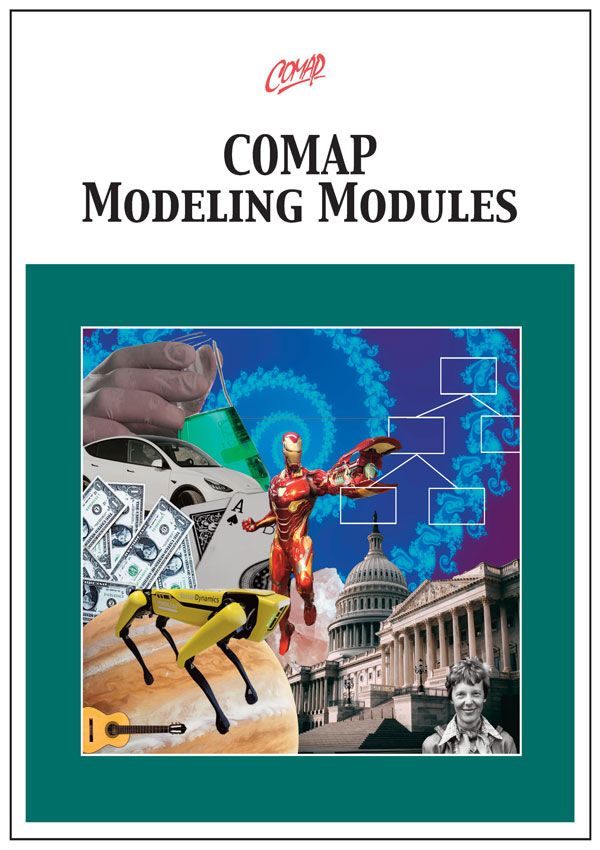
Mathematics Topics:
Application Areas:
You must have one of our Free Memberships or a paid Full Membership to download this resource.
If you're already a member, login here.